Artificial Intelligence (AI) is revolutionizing various sectors by streamlining tasks, enhancing decision-making, and improving efficiency. However, despite its potential, a critical concern has emerged — can AI be biased? The simple answer is yes. This guide delves into the causes of AI bias, its implications, and the steps that can be taken to mitigate it.
AI bias refers to the phenomenon where an artificial intelligence system produces results that are systematically prejudiced due to flawed data or biased programming. When AI models are trained on historical data, they can inadvertently learn patterns that reflect societal inequalities, prejudices, or stereotypes. This can result in discriminatory outcomes, often perpetuating existing biases rather than eliminating them.
Why Do AI Models Become Biased?
- Flawed Training Data
AI systems learn from vast datasets, which are often collected from human actions and decisions. If the data used to train these systems reflects historical inequalities, stereotypes, or prejudices, the AI model may internalize and replicate these biases. - Bias in Algorithm Design
The algorithms that drive AI systems are created by humans, and they may unintentionally encode biases through their design. Even if developers strive to make unbiased models, unconscious biases can still influence the design process. - Bias Amplification in Feedback Loops
AI systems often learn from their own decisions in an ongoing process called feedback loops. If an AI system makes biased decisions, it may continue reinforcing these biases, making the problem worse over time.
The Implications of AI Bias
AI bias can have significant and far-reaching consequences, particularly in sensitive areas such as hiring, law enforcement, and healthcare. Some of the most pressing implications include:
- Discriminatory Hiring Practices
AI recruitment tools are widely used to screen resumes and evaluate candidates. If the training data includes gender or racial biases, the AI system might favor male or white candidates, even if they are not the best fit for the position. This can further entrench inequality in the workplace. - Unfair Legal Outcomes
In law enforcement, predictive policing algorithms are used to predict where crimes are likely to occur. However, if these models are trained on biased data from over-policed neighborhoods, they may disproportionately target minority communities, leading to unfair treatment. - Healthcare Disparities
In healthcare, AI systems are increasingly being used for diagnostics and treatment recommendations. If these models are trained on data that lacks representation from diverse populations, they may fail to accurately diagnose or treat conditions in underrepresented groups, exacerbating existing healthcare disparities. - Reinforcement of Social Inequalities
AI bias can perpetuate long-standing social inequalities by reinforcing stereotypes. For example, a recommendation algorithm that predominantly shows advertisements for high-end products to affluent individuals might reinforce social class distinctions, limiting opportunities for marginalized groups.
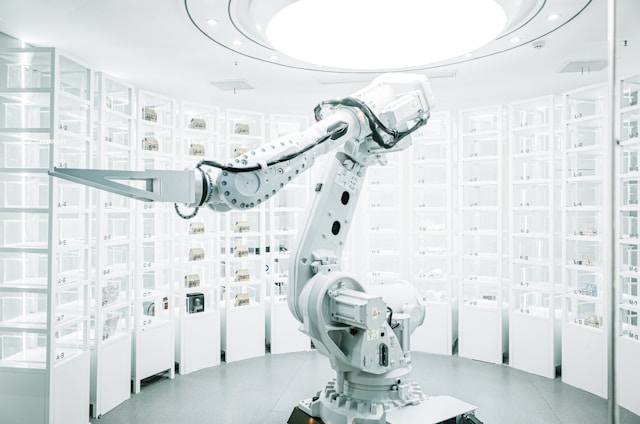
How to Tackle Bias in AI Models
Efforts are underway to identify and mitigate bias in AI models, but it requires a multi-faceted approach that involves both technical solutions and social awareness see casino en ligne france. Here are key strategies to address AI discrimination:
1. Using Diverse and Representative Data
The first step in reducing bias is ensuring that the data used to train AI models is diverse and representative of all demographic groups. This means including data from various ethnicities, genders, ages, socioeconomic backgrounds, and geographic regions. By diversifying datasets, AI models can learn to recognize and treat a broader spectrum of human experiences.
2. Regular Auditing of AI Systems
Bias in AI is not always apparent from the outset, so it is crucial to conduct regular audits of AI systems to detect and address any unintended discrimination. Auditing can help identify discrepancies in outcomes for different demographic groups and allow developers to make necessary adjustments to the model.
3. Incorporating Fairness Algorithms
Developers can integrate fairness algorithms into the training process. These algorithms are designed to reduce or eliminate bias by enforcing fairness constraints on AI models. For example, fairness algorithms can be used to ensure that predictive models do not disproportionately favor one demographic group over others.
4. Encouraging Diversity in AI Development Teams
Diverse teams bring different perspectives and experiences, which can help identify potential sources of bias that a more homogenous team might overlook. By fostering inclusivity in AI development, organizations can create more ethical and unbiased systems.
5. Transparent and Explainable AI
AI systems should be transparent and explainable, meaning their decision-making processes are understandable to humans. When AI systems provide explanations for their decisions, it becomes easier to identify biases and correct them. Explainability also increases accountability, as developers and organizations can be held responsible for biased outcomes.
6. Building AI with Ethical Guidelines
Ethical guidelines should be embedded in the development process of AI systems to ensure that fairness, equality, and justice are prioritized. Ethical frameworks can help guide the design of AI models, ensuring that they are developed with a commitment to reducing discrimination.
Conclusion
AI systems hold immense promise for improving our lives, but they are not immune to bias. As AI becomes increasingly integrated into critical areas of society, addressing discrimination in AI models is paramount. By focusing on diverse data, implementing fairness algorithms, and encouraging ethical practices, we can reduce bias and create AI systems that are fair, transparent, and just for all.
Through continuous efforts and collaboration between developers, policymakers, and communities, we can ensure that AI reaches its full potential in an equitable and inclusive manner. The future of AI is not just about technological advancement but also about creating systems that serve all people, regardless of their background or identity.